Rafael Cardoso Sampaio, Professor permanente do Programa de Pós-graduação em Ciência Política e Comunicação da Universidade Federal do Paraná (UFPR)
Maria Alejandra Nicolás, Professora do Programa de Mestrado em Políticas Públicas e Desenvolvimento da Universidade Federal da Integração Latino-Americana (UNILA)
Tainá Aguiar Junquilho, Professora do Mestrado em Direito do IDP
Luiz Rogério Lopes Silva, Professor substituto do Departamento de Ciência e Gestão da Informação da Universidade Federal do Paraná (UFPR)
Christiana Soares de Freitas, Professora dos Programas de Pós-graduação em Comunicação e em Governança e Inovação em Políticas Públicas Universidade de Brasília (UNB)
Marcio Telles, Professor permanente do Programa de Pós-Graduação em Comunicação e Linguagens da Universidade Tuiuti do Paraná (UTP)
João Senna Teixeira, Bolsista de pós-doutorado no Instituto Nacional de Ciência e Tecnologia em Democracia Digital (INCT-DD) da Universidade Federal da Bahia (UFBA)
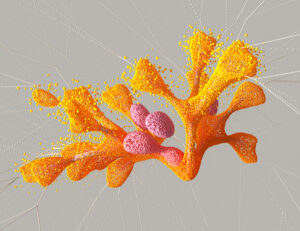
Image: Google DeepMind.
In the first part of this essay,1 we presented six main uses of Artificial Intelligence (AI) to help in various stages of the scientific endeavor; now in the second part, we discuss consequences, risks, and paradoxes in the use of AI for scientific research. Now, in this second part, we seek to present some of these risks, which arise particularly in the use of generative AI in the scientific field and in academic work. Although all the problems have not been fully mapped out, we have tried to offer initial reflections to support and encourage debate. We would like to emphasize that both essays are a summary of a larger reflection that has already been published as an article2 in SciELO Preprints.
1. Authorship and plagiarism
The trend is for AIs to become part of the development of academic texts, gradually making it difficult to differentiate between texts produced by humans or by machines. In particular, there is the unresolved question of whether a text is written by a human and only proofread by artificial intelligence, is that enough for it to stop being human? In another possibility, if a human creates a good part of a text, but then asks the AI to complement it, does this violate any ethical boundaries? If we ask the AI to read a text we have written and draft the summary or title, will it be a machine text? Bearing in mind that the human has proofread all the texts and put their name down as responsible. There is little consensus on these issues, except that artificial intelligences cannot be considered as authors of an academic paper, since they cannot be held responsible for the content produced and if any tool is used, it should be mentioned in the appropriate section of the academic paper.
As AIs can easily generate images, graphs, tables, and even entire presentations, rather than the discussion about plagiarism or “pasting”, as discussed above, it seems to us that the most fruitful discussion will be the one related to authorship, copyright, fonts, and the limits of human-machine cooperation.
It is important to note that at the time of writing this essay, no text-based AI detection tool has been evaluated as infallible or truly reliable. In fact, one study showed that it tended to have a bias against non-native English speakers. Finally, it is vital to reinforce that the large language models (LLMs) cited in this essay are NOT capable of detecting plagiarism or the use of AI, which is why we do not recommend detection applications.
2. Diminished integrity of scientific endeavor
The lack of transparency about the criteria and algorithms used by AI can lead to a lack of understanding about how decisions are made. Why did the machine recommend reading a certain text over another? Why did it recommend doing a statistical test or a specific visual representation? Moreover, it is worth noting that LLMs can occasionally produce answers that seem plausible at first glance, but on closer examination turn out to be disconnected from the context, factually incorrect or considerably distorted by the model’s biases.
As we know little or nothing about the possible unaddressed and similar biases of these models, the risk of inconsistency or inaccuracy requires a critical approach when evaluating and using its responses, underlining the importance of rigorous human supervision and meticulous review to ensure the quality and reliability of scientific writing. The use of AIs makes it difficult to trust the results and limits researchers’ ability to assess their validity and reliability, making certain aspects of the research completely unreplicable.
3. Restricted range of research possibilities
The standard established by Google, also used by academic indexers, shows a series of links to pages dealing with the requested subject based on relevance criteria.
In the new paradigm established by ChatGPT, the main output is initially a machine-generated response and only then links for further study. We will not need to go to the websites or read different opinions but have them summarized by artificial intelligence directly in the chat while proceeding the questions, reducing access to dissent, and crystallizing the most “relevant” positions, which could have effects on increasing inequalities in producing scientific knowledge.
This situation is likely to get even worse, as these artificial intelligences, despite being trained on huge databases, primarily index North American, Anglo-Saxon, and European output, especially when we are talking about academic AIs. Therefore, they will tend to reinforce a very particular view of science, which includes certain types of methods and forms of analysis that could become even more the standard for scientific research. This could have especially damaging effects on humanities research or on all qualitative research in general.
4. The paradox of knowledge production
The automation of various tasks and the outsourcing of important choices to artificial intelligences could foster a generation of future researchers who will know fewer authors and sources and will have even less knowledge of academic literature and the basis of research and programming methods.
Thus, we can easily imagine future researchers who will “read” dozens of articles simultaneously; draw up automated and substantive “literature reviews”; scrape, clean, and analyze massive amounts of data; use such models and technologies to test the limits of human knowledge and, we suppose, even achieve more substantive and innovative results. Paradoxically, we will have “less trained”, “less skilled” researchers who will nevertheless be able to carry out faster and more meaningful tasks.
Finally, we recognize that all this can generate a gigantic amount of worthless academic trash, with a tide of poorly written scientific texts, lacking in novelty, criteria, and rigor, seeking only to feed predatory journals and the CVs of students and professors in search of funding.
5. Center-periphery relations and data colonialism
Besides breaking down language barriers in terms of reading and writing, the technology also democratizes the role of research assistants, who were only available to renowned researchers from institutions in the global north. With AI tools, researchers can perform tasks such as note-taking, citation archiving, manuscript preparation, manuscript editing, form filling, audio transcription, email writing, topic list creation, text translation, and presentation creation – tasks normally relegated to assistants. This could reduce the gap in output and publication capacity between researchers from the center and the periphery of the academic world.
Even so, many of these tools are paid for, and in dollars or euros, limiting access to them for researchers from the global south. Another discussion is whether or not university resources should go towards the use of these applications and AI platforms. While this may seem strange at the moment, it is worth remembering that we have already normalized payment for servers (Microsoft, Amazon etc.), software (NVivo, Atlas.ti, Stata, SPSS, Endnote, Office etc.) and for access to certain article platforms.
Considering that research resources in the global south will continue to be lower and that the centers will pay for or develop their own AIs, the danger lies not in a decrease, but in an increase in the research gap between the center and the periphery. There is a risk that researchers from the global south will increase their dependence on technologies developed by the global north, reinforcing the issue of data colonialism, which already exists on social media platforms.
However, we point out a paradox in terms of research internationalization. We may be overly dependent on the technologies created by this center, but the number of applications dedicated to language translation and correction has increased in quantity and quality, reducing the biggest obstacle to publication in the most important journals. Apps like DeepL already deliver considerably better translations than Google Translate and we have all the aforementioned English correction tools like Grammarly, Quillbot, Writefull and the like. And all LLMs without exception are able to refine texts to be closer to standard academic text.
We are not advocating an end to translation or correction by humans, just that there will be new tools for researchers to write directly in other languages and publish in the main journals.
All of these reflections are very premature, but they seek to move away from the exclusive discussion about plagiarism and the falsification of tests by students, seeking to show that the changes will be much more significant than we are discussing. Discussion is therefore vital at this time.
The “ChatGPT and other AIs will transform all scientific research: initial reflections on uses and consequences” series consists of two posts
- ChatGPT and other AIs will transform all scientific research: initial reflections on uses and consequences – part 1
- ChatGPT and other AIs will transform all scientific research: initial reflections on uses and consequences – part 2
Notes
1. SAMPAIO, R.C., et al. ChatGPT and other AIs will transform all scientific research: initial thoughts on uses and consequences – part 1 [online]. SciELO in Perspective, 2023 [viewed 14 November 2023]. Available from: https://blog.scielo.org/en/2023/11/10/chatgpt-and-other-ais-will-transform-all-scientific-research-initial-thoughts-on-uses-and-consequences-part-1/
2. SAMPAIO, R.C., et al. ChatGPT and other AIs will change all scientific research: initial reflections on uses and consequences. SciELO Preprints [online]. 2023 [viewed 14 November 2023]. https://doi.org/10.1590/SciELOPreprints.6686. Available from: https://preprints.scielo.org/index.php/scielo/preprint/view/6686/version/7074
References
MOHL, P. Seeing threats, sensing flesh: human–machine ensembles at work. AI & Society [online]. 2020, vol.36, pp.1243-1252 [viewed 14 November 2023]. https://doi.org/10.1007/s00146-020-01064-1. Available from: https://link.springer.com/article/10.1007/s00146-020-01064-1
SAMPAIO, R.C., et al. ChatGPT and other AIs will change all scientific research: initial reflections on uses and consequences. SciELO Preprints [online]. 2023 [viewed 14 November 2023]. https://doi.org/10.1590/SciELOPreprints.6686. Available from: https://preprints.scielo.org/index.php/scielo/preprint/view/6686/version/7074
SAMPAIO, R.C., et al. ChatGPT and other AIs will transform all scientific research: initial thoughts on uses and consequences – part 1 [online]. SciELO in Perspective, 2023 [viewed 14 November 2023]. Available from: https://blog.scielo.org/en/2023/11/10/chatgpt-and-other-ais-will-transform-all-scientific-research-initial-thoughts-on-uses-and-consequences-part-1/
External links
DeepL Translate: https://www.deepl.com/translator
Google Translate: https://translate.google.com
Grammarly: https://www.grammarly.com/
QuillBot: https://quillbot.com/
SciELO Preprints: https://preprints.scielo.org/
Writefull: http://writefull.com/
Translated from the original in Portuguese by Lilian Nassi-Calò.
Como citar este post [ISO 690/2010]:
Recent Comments