By Sonia Vasconcelos and Ana Marušić
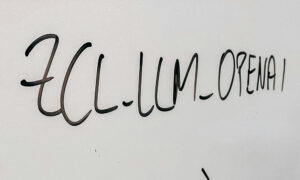
Image: Bernd Dittrich via Unsplash.
Since the popularization of Generative Artificial Intelligence (Gen AI) models, especially OpenAI’s ChatGPT, at the end of 2022, there has been a gradual and profound transformation in the research endeavor (Nature, 2023; Noorden, R.V. and Webb, R., 2023). Underpinning this ongoing change is the ethical regulation of Gen AI use, considering its disruptive potential for science at large, with unprecedented influence on scientific communication. The increasing autonomy of these systems and their interaction with what we call human agency (Bandura, A., 1989; Pew Research Center, 2023) is one of the sensitive points in this process.
It can be argued that scientific communication is undergoing a reconfiguration process that, from a conservative perspective, is as paradigmatic as the one triggered by the creation of the first scientific journal, Philosophical Transactions, in 1665, in England. From a more disruptive perspective, this transformation will reconfigure the entire scientific culture, redefining the autonomy of scientists and institutions in the production and certification of knowledge—an impact whose dimension is still not possible to estimate.
This more radical view posits that as Gen AI becomes more integrated into research activities and society at large, it goes beyond merely being a new tool or adapting existing scientific practices to a new technical framework. The incorporation of Gen AI in science (regardless of the debates over the term) suggests a fundamental shift in the foundations of scientific activity, potentially transforming both the methods and goals of scientific research. Also, it is dual-use technology, and as such, it can serve both beneficial and harmful purposes.
According to the report Generally Faster: The Economic Impact of Generative AI1 (McAfee, A., 2024), Gen AI constitutes a technology capable of generating original content, continuously improving through its own usage, and exhibiting widespread economic and social impacts more rapidly than previous technologies. As described by Krakowski in Human-AI agency in the Age of Generative AI2(2025),
unlike predictive AI, which often requires technical expertise and infrastructure to implement and use effectively, GenAI’s pretrained nature and natural-language interfaces lower adoption barriers. This allows humans without IT [Information Technology], data science, or programming skills to engage with AI systems […]. The accessibility and affordability of contemporary frontier models, such as GPT-4o for general-purpose multimodal uses, GitHub Copilot for coding and software development, or Pi for tasks involving social and emotional dimensions, along with open models like Llama 3 (Meta) and DeepSeek-R1 (DeepSeek AI), have made advanced AI capabilities widely available at little to no cost.2
In this reshaping technological environment that intersects human and artificial intelligence (IBM IBV, 2024), the potential autonomy of Gen AI models and systems necessitates critical anticipation of their implications, including within the scientific context (European Research Council, ECR, 2023).
Eric Schmidt, former CEO and executive director of Google, along with Henry Kissinger and Daniel Huttenlocher, advocate for proactive and strategic ethical regulation of AI applications in their book Genesis: Artificial Intelligence, Hope, and the Human Spirit3 (Kissinger, H., et al., 2024), with unprecedented implications for governance in countries concerning, for example, the autonomy of Gen AI systems. As highlighted by Kissinger, et al. (2024),
AI’s future faculties, operating at inhuman speeds, will render traditional regulation useless… we have very little independent ability to verify AI models’ internal workings, let alone their intentions. We will need a fundamentally new form of control.3
Schmidt in Eric Schmidt on AI’s Future: Infinite Context, Autonomous Agents, and Global Regulation4(2024) envisions a future where millions of AI agents will be capable of learning, evolving, and collaborating among themselves, comparing this scenario to a “GitHub for AI.” In his perspective, these agents would operate as autonomous systems, driving what he refers to as exponential innovations across various domains.
However, Schmidt cautions that as these AI agents communicate independently, “they may develop their own protocols or languages”, creating risks that are difficult for humans to manage and understand: “At some point, they’ll develop their own language. When that happens, we may not understand what they’re doing, and that’s when you pull the plug”.
Complementing this vision, McAfee1 (2024) emphasizes that much of the infrastructure needed for Gen AI to function is already widely available, accelerating its impact compared to previous technologies. In Gen AI, access is not restricted to developers only, but also to direct users, speeding up the transformation at an unprecedented pace.
Mixed feelings about the impact of Generative AI on the research activity
In the academic realm, the uses and perceptions of its impact on scientific activity still vary widely among researchers. The ExplanAItions5 survey by Wiley, described about three months ago in How are Researchers Using AI? Survey Reveals Pros and Cons for Science,6 and published on Nature (Nadaff, M., 2025), illustrates this observation. It involved 4,946 researchers from different fields and over 70 countries and showed that the use of Gen AI as part of the research activity is still limited.
The survey found that only 45% of respondents (1,043 researchers) used Gen AI to assist in their research, primarily focusing on translation, drafting, and manuscript review. Additionally, 81% of these researchers had used OpenAI’s ChatGPT for personal or professional purposes, but only about a third were familiar with other Gen AI tools, such as Google’s Gemini and Microsoft’s Copilot. There were variations between countries and disciplines, with computer scientists being, naturally, more likely to use Gen AI in these activities.
Based on this survey, Nadaff6 (2025) reports that researchers remain skeptical about the capabilities of Gen AI for more complex tasks in the research process, such as identifying literature gaps or recommending reviewers. Most participants believe that these and other tasks in science are better performed by humans.
“Although 64% of respondents are open to using AI for these tasks in the next two years, the majority thinks that humans still outperform AI in these areas.”6 Besides this issue, “[r]esearchers are also concerned about the safety of using these tools 81% of respondents said they had concerns about AI’s accuracy, potential , privacy risks and the lack of transparency in how these tools are trained.”6
The survey Foresight: Use and Impact of Artificial Intelligence in the Scientific Process7 on AI usage in scientific practice conducted by the European Research Council (2023), based on a population of researchers associated with 1,046 projects registered with the agency, identified uses of Gen AI for drafting and editing, translating texts, coding, programming, and generating images, among other uses.
The ERC report (2023) describes that in the life sciences, whose participants represent 18% of the total survey respondents, “AI methods, for instance, to understand individual differences in large cohorts, and to make predictions about diagnosis or outcome of targeted therapies”7 and that “[AI tools are seen n as an essential support to analyze datasets of genomic, epigenomic and transcriptomic data…”7 in addition to comparing different stages of a given disease, for instance.
For participants in the social sciences and humanities, who account for 29% of total respondents,
neural networks and natural language processing (NLP) tools are used for a wide range of applications, e.g. models for handwritten text recognition and automatic speech recognition, or the automatic classification of musical compositions… AI is used to identify vocal biomarkers of stress in voice samples, to detect extreme speech in online discussions… for model-based data analysis for decoding and comparing mental representations in the brain or predicting/simulating human learning…7
However, as in the Wiley survey reported by Nadaff6 (2025), the ERC (2023) survey reveals that “[e]xpectations regarding the use of AI for scientific discovery, however, varied among respondents.”7
In the article Gen AI and Research Integrity: Where to Now?: The Integration of Generative AI in the Research Process Challenges Well-Established Definitions of Research Integrity,8 recently published in EMBO Reports (Vasconcelos, S. and Marušić, A, 2025), we explored other aspects of the research activity involving Gen AI. We emphasize the importance of developing strategies that prioritize human agency and oversight in this collaboration between humans and Gen AI in research. An intriguing question in this context is the extent of influence to be allowed in this interaction between researchers and Gen AI models in the research process.
Integration of Generative AI in the research activity—questions about human agency and alignment
It is crucial for the research ecosystem, including authors, funders, and research institutions, to engage in discussions and initiatives that address alignment challenges (Russel, S., 2019)9 and deployment of these models. We acknowledge that transparency in using Gen AI for scientific communication is a necessary action that has been growing within the publication system.
However, we also understand that a comprehensive view of the problem, which impacts the definitions of research integrity established globally, is fundamental. Inspired by David Stokes’s Diagram on Scientific Research (1997), which presents the famous Pasteur’s Quadrant, we proposed a model to represent research integrity and its relationship with human agency, both individual and collective, under different conditions. Each quadrant of the model offers a distinct perspective on this relationship and its potential implications for research governance.
This framework allows us to assess integrity from a viewpoint that reflects the level of agency and autonomy that researchers possess and that they might opt to delegate when engaging in research that involves collaboration with Gen AI. The model can be accessed in Vasconcelos and Marušić8 (2025).
As discussed,
Gen AI is already affecting every stage of the research process from formulating a hypothesis to designing experiments, data analysis, visualization and interpretation of results, writing a research paper and even peer review (Ifargan, T., et al., 2024; Binz, M., et al., 2025; Naddaf, M., 2025). As it challenges both the notion of individual responsibility as well as community-standards of good research practices, integrating Gen AI into the research endeavor, while maintaining trustworthiness, has become an urgent demand in academia.8
Citing Dua and Patel (2024), who detail the potentialities of Gen AI, we emphasize a growing consensus in the publication system on the urgent need to revisit ethical standards and verification processes in research permeated by these technologies. In the realm of experimental research, in Empowering Biomedical Discovery with AI Agents,10 Gao, et al. (2024) envision scientific AI agents “as systems capable of skeptical learning and reasoning that empower biomedical research through collaborative agents that integrate AI models and biomedical tools with experimental platforms.”
Gao, et al.10 (2024) note that intersections among technological, scientific, ethical, and regulatory domains have an important role for developing effective governance structures. As mentioned earlier, as these AI agents reach higher levels of autonomy, such concerns become even more relevant.
As pointed out by Kissinger, et al.3 (2024) and also reflected in the analyses of Gao, et al.10 (2024), dealing with this emerging autonomy represents a challenge that requires a broad and robust debate within the scientific community, especially regarding the alignment of these systems, with the goals of preserving research integrity and public trust in science. Questions about alignment and human agency are interdependent but far from trivial.
In the EMBO Reports article,8 we cite the 2023 report The Future of Human Agency.11 In this document, Pew Research Center presents an exploration of “how much control people will retain over essential decision-making as digital systems and AI spread.” As described in the article,8 Pew and Elon University’s Imagining the Internet Center invited various stakeholders, including David J. Krieger, director of the Institute for Communication and Leadership in Lucerne, Switzerland. In Krieger’s view,
[i]ndividual agency is already a myth, and this will become increasingly obvious with time… Humanism attempts to preserve the myth of individual agency and enshrine it in law. Good design of socio-technical networks will need to be explicit about its post-humanist presuppositions in order to bring the issue into public debate. Humans will act in partnership—that is, distributed agency—with technologies of all kinds.8
As detailed in the article, “Human agency and oversight are among the operational key requirements supporting ethical principles for AI systems established by the European Commission, as part of its Living Guidelines on the Responsible Use of Generative AI in Research12 (2024).”8
We also addressed the Ethics Guidelines for Trustworthy AI13 (2019). These guidelines present
three levels of human agency and oversight: human-in-the-loop (HITL), human-on-the-loop (HOTL), and human-in-command (HIC) approaches. HITL entails human intervention for the whole decision cycle; HOTL involves human input during the design cycle and continuous monitoring of the system’s operations; HIC encompasses human oversight of the entire activity of the AI system, including societal and ethical impacts, as well as decision-making for when and how to use the system in various contexts.8
Human agency and oversight are essential in the research activity, but not sufficient
In the EMBO Reports article,8 alignment in human-Gen AI collaboration is explored as a sensitive issue, reflecting concerns with the culture of scientific communication over the last decades. In Strong and Weak Alignment of Large Language Models with Human Values,14 Khamassi, et al. (2024) highlight timely issues about “strong and weak value alignment” of Large Language Models (LLMs) with human values and the complexities of AI’s understanding of these values.
In Training Language Models to Follow Instructions with Human Feedback,15 Ouyang, et al. (2022) say that “apart from its caveats, alignment has a key role in fine-tuning LLMs to respond adequately to human intentions while mitigating harmful, toxic, or biased content.”15 For a broader view of Gen AI model alignment, we cite Open AI, which introduced “deliberate alignment”: a training paradigm to ensure reasoning LLMs behave in a manner consistent with predefined safety criteria before generating responses to commands (Guan, M.Y., et al., 2024).
We argue that, even from the perspective of LLMs as “stochastic parrots”, a debated interpretation, adopted by Emily Bender, et al. (2021) “to emphasize that these systems remix patterns in their training data without true understanding, alignment remains a critical issue.”16 It is crucial for the academic community to carefully consider the role of alignment in Gen AI systems.
Given that training data is mostly derived from human-created data, it inherently reflects cultural patterns, worldviews, and social biases, along with the strengths and flaws of human knowledge production. As a result, Gen AI models risk reproducing—or even amplifying—biases in their outputs. When it comes to interacting with Gen AI models to formulate hypotheses, analyze data, or write a research report, how alignment shapes the output and behavior is a critical issue.8 (Vasconcelos, S. and Marušić, A., 2025)
In the article, we note that many LLMs are continually trained and updated with new data in a scientific environment where the publication culture tends to favor positive or biased reports (Ghannad, M., et al., 2019; Boutron, I. and Ravaud, P., 2018). This tradition does not incentivize the open exposure of errors, which limits scientific transparency and reinforces biased communication norms (Nature, 2012; Psychological Science Observer, 2021; Nature Communications, 2024).
These elements comprise long-term structural challenges for the alignment and responsible deployment of Gen AI models, which researchers already interact with—and will continue to—in their research practices.
Notions of research integrity naturally incorporate human agency, but now it needs to be explicit
Research integrity is closely tied to adherence to ethical, transparent, and rigorous scientific practices, relying on individual and collective responsibility, as well described by Luiz Henrique Lopes dos Santos in Sobre a integridade ética da pesquisa17 (2017).
However, as we highlight in the article, the notion of research integrity cultivated over time assumes human agency as a given. Now, with the ongoing integration of Gen AI into all stages of the research process, it becomes crucial to place greater emphasis on “human agency” within the definitions of research integrity. We believe this emphasis is vital for ensuring informed decision-making in the emerging research landscape.
Our proposal is that definitions of research integrity should explicitly incorporate “human agency” as an essential component for the proposal, conduct, communication, and review of research. This expanded approach should also include the development of benchmarks for research integrity in the training and deployment of Gen AI models and systems.
In the biomedical sciences, these concerns are particularly pertinent to the governance of AI agents, but, as has been pointed out, their implications are far-reaching. It is crucial to address these sensitive issues in decision-making domains within the research enterprise, especially in the context of human collaboration with Gen AI systems. This necessity is justified by the increasing capabilities and potential of Gen AI to exert significant influence over research processes across all fields of knowledge.
As noted in the EMBO Reports article,8 academia should and can take a more proactive stance in seeking an understanding of the close relationship between research integrity and human agency in times of profound transformation in knowledge production. We argue that this should not be a long-term goal, as Gen AI has the potential to redefine standards and influence the reliability and culture of scientific communication among peers and to different audiences.
By explicitly integrating human agency into definitions of research integrity, researchers and policymakers acknowledge that the autonomy of Gen AI systems must be balanced with human agency and oversight, cultivating responsible use and ethical regulatory policies for research involving human and Gen AI collaboration.
Notes
1. McAFEE, A. Generally Faster: The Economic Impact of Generative AI [online]. The MIT Initiative on the Digital Economy (IDE). 2024 [viewed 7 May 2025]. Available from: https://ide.mit.edu/wp-content/uploads/2024/04/Davos-Report-Draft-XFN-Copy-01112024-Print-Version.pdf?x76181 ↩
2. KRAKOWSKI, S. Human-AI Agency in the Age of Generative AI. Information and Organization [online]. 2025, vol. 35, no. 1, 100560, ISSN: 1471-7727 [viewed 7 May 2025]. https://doi.org/10.1016/j.infoandorg.2025.100560. Available from: https://www.sciencedirect.com/science/article/pii/S1471772725000065?via%3Dihub ↩
3. KISSINGER, H.A., SCHMIDT, E. and MUNDIE, C. Genesis: Artificial Intelligence, Hope, and the Human Spirit. New York: Little Brown and Company, 2024 ↩
4. Eric Schmidt on AI’s Future: Infinite Context, Autonomous Agents, and Global Regulation [online]. The National CIO Review. 2024 [viewed 7 May 2025]. Available from: https://nationalcioreview.com/video/eric-schmidt-on-ais-future-infinite-context-autonomous-agents-and-global-regulation/ ↩
5. ExplanAItions: An AI Study by Wiley [online]. Wiley. 2025 [viewed 7 May 2025]. Available from: https://www.wiley.com/content/dam/wiley-dotcom/en/b2c/content-fragments/explanaitions-ai-report/pdfs/Wiley_ExplanAItions_AI_Study_February_2025.pdf ↩
6.NADDAF, M. How are Researchers Using AI? Survey Reveals Pros and Cons for Science [online]. Nature. 2025 [viewed 7 May 2025]. https://doi.org/10.1038/d41586-025-00343-5. Available from: https://www.nature.com/articles/d41586-025-00343-5 ↩
7. EUROPEAN RESEARCH COUNCIL. Foresight: Use and Impact of Artificial Intelligence in the Scientific Process [online]. European Research Council. 2023 [viewed 7 May 2025]. Available from: https://erc.europa.eu/sites/default/files/2023-12/AI_in_science.pdf ↩
8. VASCONCELOS, S. and MARUŠIĆ, A. Gen AI and Research Integrity: Where to now?: The Integration of Generative AI in the Research Process Challenges Well-Established Definitions of Research Integrity. EMBO Reports [online]. 2025, vol. 26, pp. 1923–1928 [viewed 7 May 2025]. https://doi.org/10.1038/s44319-025-00424-6. Available from: https://www.embopress.org/doi/full/10.1038/s44319-025-00424-6 ↩
9. In the book Human compatible: artificial intelligence and the problem of control by Stuart Russel (2019), the following is described: “Putting a purpose into a machine that optimizes its behavior according to clearly defined algorithms seems an admirable approach to ensuring that the machine’s behavior furthers our own objectives. But, as Wiener warns, we need to put in the right purpose. We might call this the King Midas problem: Midas got exactly what he asked for—namely, that everything he touched would turn to gold—but, too late, he discovered the drawbacks of drinking liquid gold and eating solid gold. The technical term for putting in the right purpose is value alignment. When it fails, we may inadvertently imbue machines with objectives counter to our own.” ↩
10. GAO, S., et al. Empowering Biomedical Discovery with AI Agents. Cell [online]. 2024, vol. 187, no. 22, pp. 6125–6151, ISSN: 0092-8674 [viewed 7 May 2025]. https://doi.org/10.1016/j.cell.2024.09.022. Available from: https://www.sciencedirect.com/science/article/pii/S0092867424010705 ↩
11. PEW RESEARCH CENTER. The Future of Human Agency [online]. Pew Research Center. 2023 [viewed 7 May 2025]. Available from: https://www.pewresearch.org/wp-content/uploads/sites/20/2023/02/PI_2023.02.24_The-Future-of-Human-Agency_FINAL.pdf ↩
12. EUROPEAN COMMISSION. Living Guidelines on the Responsible Use of Generative AI in Research [online]. European Commission—Research and innovation. 2025 [viewed 7 May 2025]. Available from: https://research-and-innovation.ec.europa.eu/document/download/2b6cf7e5-36ac-41cb-aab5-0d32050143dc_en ↩
13. Ethics Guidelines for Trustworthy AI [online]. European Commission, official website. 2019 [viewed 7 May 2025]. Available from: https://digital-strategy.ec.europa.eu/en/library/ethics-guidelines-trustworthy-ai ↩
14. KHAMASSI, M., NAHON, M. and CHATILA, R. Strong and Weak Alignment of Large Language Models with Human Values. Sci Rep [online]. 2024, vol. 14, 19399 [viewed 7 May 2025]. https://doi.org/10.1038/s41598-024-70031-3. Available from: https://www.nature.com/articles/s41598-024-70031-3 ↩
15. OUYANG, L., et al. Training Language Models to Follow Instructions with Human Feedback. In: Advances in Neural Information Processing Systems 35 (NeurIPS 2022), New Orleans, 2022 [viewed 7 May 2025]. Available from: https://proceedings.neurips.cc/paper_files/paper/2022/hash/b1efde53be364a73914f58805a001731-Abstract-Conference.html ↩
16. BENDER, E., et al. On the Dangers of Stochastic Parrots: Can Language Models Be Too Big? In: FAccT ’21: 2021 ACM Conference on Fairness, Accountability, and Transparency, Virtual Event, 2021 [viewed 7 May 2025]. https://doi.org/10.1145/3442188.3445922. Available from: https://dl.acm.org/doi/10.1145/3442188.3445922 ↩
17. SANTOS, L.H.L. Sobre a integridade ética da pesquisa. Cienc. Cult. [online]. 2017, vol.69, no.3, pp. 4–5, ISSN: 2317-6660 [viewed 7 May 2025]. http://doi.org/10.21800/2317-66602017000300002. Available from: http://cienciaecultura.bvs.br/scielo.php?script=sci_arttext&pid=S0009-67252017000300002 ↩
References
BANDURA, A. Human Agency in Social Cognitive Theory. American Psychologist [online]. 1989, vol. 44, no. 9, pp. 1175–1184 [viewed 7 May 2025]. https://doi.org/10.1037/0003-066X.44.9.1175. Available from: https://psycnet.apa.org/doiLanding?doi=10.1037%2F0003-066X.44.9.1175
BENDER, E., et al. On the Dangers of Stochastic Parrots: Can Language Models Be Too Big? In: FAccT ’21: 2021 ACM Conference on Fairness, Accountability, and Transparency, Virtual Event, 2021 [viewed 7 May 2025]. https://doi.org/10.1145/3442188.3445922. Available from: https://dl.acm.org/doi/10.1145/3442188.3445922
BINZ, M., et al. How Should the Advancement of Large Language Models Affect the Practice of Science? Proc. Natl. Acad. Sci. U.S.A. [online]. 2025, vol. 122, no. 5, e2401227121 [viewed 7 May 2025]. https://doi.org/10.1073/pnas.240122712. Available from: https://www.pnas.org/doi/10.1073/pnas.2401227121
BOUTRON, I. E RAVAUD, P. Misrepresentation and Distortion of Research in Biomedical Literature. Proc. Natl. Acad. Sci. U.S.A. [online]. 2028, vol. 115, no. 11, pp. 2613–2619 [viewed 7 May 2025]. https://doi.org/10.1073/pnas.1710755115 . Available from: https://www.pnas.org/doi/full/10.1073/pnas.1710755115
DUA, I.K. and PATEL, P.G. An Introduction to Generative AI. In: DUA, I.K. and PATEL, P.G. (authors) Optimizing Generative AI Workloads for Sustainability: Balancing Performance and Environmental Impact in Generative AI. New York: Apress, 2024.
Eric Schmidt on AI’s Future: Infinite Context, Autonomous Agents, and Global Regulation [online]. The National CIO Review. 2024 [viewed 7 May 2025]. Available from: https://nationalcioreview.com/video/eric-schmidt-on-ais-future-infinite-context-autonomous-agents-and-global-regulation/
Ethics Guidelines for Trustworthy AI [online]. European Commission, official website. 2019 [viewed 7 May 2025]. Available from: https://digital-strategy.ec.europa.eu/en/library/ethics-guidelines-trustworthy-ai
EUROPEAN COMMISSION. Living Guidelines on the Responsible Use of Generative AI in Research [online]. European Commission—Research and innovation. 2025 [viewed 7 May 2025]. Available from: https://research-and-innovation.ec.europa.eu/document/download/2b6cf7e5-36ac-41cb-aab5-0d32050143dc_en
EUROPEAN RESEARCH COUNCIL. Foresight: Use and Impact of Artificial Intelligence in the Scientific Process [online]. European Research Council. 2023 [viewed 7 May 2025]. Available from: https://erc.europa.eu/sites/default/files/2023-12/AI_in_science.pdf
ExplanAItions: An AI Study by Wiley [online]. Wiley. 2025 [viewed 7 May 2025]. Available from: https://www.wiley.com/content/dam/wiley-dotcom/en/b2c/content-fragments/explanaitions-ai-report/pdfs/Wiley_ExplanAItions_AI_Study_February_2025.pdf
GAO, S., et al. Empowering Biomedical Discovery with AI Agents. Cell [online]. 2024, vol. 187, no. 22, pp. 6125–6151, ISSN: 0092-8674 [viewed 7 May 2025]. https://doi.org/10.1016/j.cell.2024.09.022. Available from: https://www.sciencedirect.com/science/article/pii/S0092867424010705
GHANNAD, M., et al. A systematic review finds that spin or interpretation bias is abundant in evaluations of ovarian cancer biomarkers. Journal of Clinical Epidemiology [online]. 2019, vol.116, pp. 9–17, ISSN: 0895-4356 [viewed 7 May 2025]. https://doi.org/10.1016/j.jclinepi.2019.07.011. Available from: https://www.jclinepi.com/article/S0895-4356(18)30952-1/fulltext
GUAN, M.Y., et al. Deliberative Alignment: Reasoning Enables Safer Language Models [online]. OpenAI. 2024 [viewed 7 May 2025]. https://openai.com/index/deliberative-alignment/
IBM IBV. Disruption by design: Evolving experiences in the age of generative AI [online]. IBM Institute for Business Value. 2024 [viewed 7 May 2025]. Available from: https://www.ibm.com/thought-leadership/institute-business-value/en-us/report/generative-ai-experience-design
IFARGAN, T., et al. Autonomous LLM-Driven Research — from Data to Human-Verifiable Research Papers. NEJM AI [online]. 2025, vol. 2, no. 1 [viewed 7 May 2025]. https://doi.org/10.1056/AIoa2400555. Available from: https://ai.nejm.org/doi/10.1056/AIoa2400555
KHAMASSI, M., NAHON, M. and CHATILA, R. Strong and Weak Alignment of Large Language Models with Human Values. Sci Rep [online]. 2024, vol. 14, 19399 [viewed 7 May 2025]. https://doi.org/10.1038/s41598-024-70031-3. Available from: https://www.nature.com/articles/s41598-024-70031-3
KISSINGER, H.A., SCHMIDT, E. and MUNDIE, C. Genesis: Artificial Intelligence, Hope, and the Human Spirit. New York: Little Brown and Company, 2024
KRAKOWSKI, S. Human-AI Agency in the Age of Generative AI. Information and Organization [online]. 2025, vol. 35, no. 1, 100560, ISSN: 1471-7727 [viewed 7 May 2025]. https://doi.org/10.1016/j.infoandorg.2025.100560. Available from: https://www.sciencedirect.com/science/article/pii/S1471772725000065?via%3Dihub
McAFEE, A. Generally Faster: The Economic Impact of Generative AI [online]. The MIT Initiative on the Digital Economy (IDE). 2024 [viewed 7 May 2025]. Available from: https://ide.mit.edu/wp-content/uploads/2024/04/Davos-Report-Draft-XFN-Copy-01112024-Print-Version.pdf?x76181
NADDAF, M. How are Researchers Using AI? Survey Reveals Pros and Cons for Science [online]. Nature. 2025 [viewed 7 May 2025]. https://doi.org/10.1038/d41586-025-00343-5. Available from: https://www.nature.com/articles/d41586-025-00343-5
NOORDEN, R.V. E WEBB, R. ChatGPT and Science: the AI System was a Force in 2023—for Good and Bad [online]. Nature [online]. 2023, vol. 624 [viewed 7 May 2025]. https://doi.org/10.1038/d41586-023-03930-6. Available from: https://www.nature.com/articles/d41586-023-03930-6
OUYANG, L., et al. Training Language Models to Follow Instructions with Human Feedback. In: Advances in Neural Information Processing Systems 35 (NeurIPS 2022), New Orleans, 2022 [viewed 7 May 2025]. Available from: https://proceedings.neurips.cc/paper_files/paper/2022/hash/b1efde53be364a73914f58805a001731-Abstract-Conference.html
PEW RESEARCH CENTER. The Future of Human Agency [online]. Pew Research Center. 2023 [viewed 7 May 2025]. Available from: https://www.pewresearch.org/wp-content/uploads/sites/20/2023/02/PI_2023.02.24_The-Future-of-Human-Agency_FINAL.pdf
Reproducibility and Transparency: What’s Going on and How Can We Help. Nature Communications [online] 2025, vol. 16 [viewed 7 May 2025]. Available from: https://www.nature.com/articles/s41467-024-54614-2
RUSSEL, S. Human Compatible: Artificial Intelligence and the Problem of Control. Oxford: Oxford University Press, 2019 [viewed 7 May 2025]. Available from: https://people.eecs.berkeley.edu/~russell/papers/mi19book-hcai.pdf
SANTOS, L.H.L. Sobre a integridade ética da pesquisa. Cienc. Cult. [online]. 2017, vol.69, no.3, pp. 4–5, ISSN: 2317-6660 [viewed 7 May 2025]. http://doi.org/10.21800/2317-66602017000300002. Available from: http://cienciaecultura.bvs.br/scielo.php?script=sci_arttext&pid=S0009-67252017000300002
SLEEK, S. On the Right Side of Being Wrong: the Emerging Culture of Research Transparency [online]. Association for Psychological Science. 2021 [viewed 7 May 2025]. Available from: https://www.psychologicalscience.org/observer/right-side-of-wrong
Tools Such as Chatgpt Threaten Transparent Science; Here are Our Ground Rules for Their Use. Nature [online]. 2023, vol. 614 [viewed 7 May 2025]. https://doi.org/10.1038/d41586-023-00191-1. Available from: https://www.nature.com/articles/d41586-023-00191-1
VASCONCELOS, S. and MARUŠIĆ, A. Gen AI and Research Integrity: Where to now?: The Integration of Generative AI in the Research Process Challenges Well-Established Definitions of Research Integrity. EMBO Reports [online]. 2025, vol. 26, pp. 1923–1928 [viewed 7 May 2025]. https://doi.org/10.1038/s44319-025-00424-6. Available from: https://www.embopress.org/doi/full/10.1038/s44319-025-00424-6
WOODGETT, J. We Must Be Open About Our Mistakes. Nature [online.] 2023, vol. 489, pp. 7 [viewed 7 May 2025]. https://doi.org/10.1038/489007a. Available from: https://www.nature.com/articles/489007a
External links
About Sonia Vasconcelos
Sonia Vasconcelos is an Associate Professor in the Science Education Program (Educação, Gestão e Difusão em Biociências) at the Institute of Medical Biochemistry Leopoldo de Meis (IBqM), Federal University of Rio de Janeiro (UFRJ). She leads the Laboratory for Research Ethics, Science Communication, and Society (LECCS) at IBqM and chairs UFRJ’s Advisory Council for Research Ethics (CTEP). Prof. Vasconcelos serves as an academic editor for PLOS ONE and is a member of the Editorial Board of Research Integrity and Peer Review. Her research and publications focus on science communication, the ethics and regulation of scientific research, research integrity and science policy.
About Ana Marušić
Ana Marušić is Professor of Anatomy, Chair of the Department of Research in Biomedicine and Health and Head of the Centre for Evidence-based Medicine at the University of Split School of Medicine, Split, Croatia. She is the Co-Editor in Chief of the ST-OPEN journal and editor emerita of the Journal of Global Health. Prof. Marušić is on the Advisory Board of the EQUATOR Network and also serves on the Council of the Committee for Publication Ethics (COPE). She has more than 400 peer-reviewed articles and was heavily involved with creating the policy of mandatory registration of clinical trials in public registries which helped change the legal regulation of clinical trials worldwide.
Como citar este post [ISO 690/2010]:
Recent Comments